Background – A challenge in developing AI models for Tumor-infiltrating lymphocytes (TIL) detection is the lymphocyte ground truth (GT), typically obtained by manual labeling of each cell on H&E, which suffers from human variability. We show that a consensus of pathologists can result in a systematic under-labeling, but IHC-derived GT (cells on H&E slides labeled with IHC-derived lymphocyte labels), contains more labeled cells. A previously developed lymphocyte model has substantially higher performance on IHC-derived GT than H&E-only GT.
Design – H&E stained WSIs from metastatic NSCLC tumors were collected for 2 datasets, one annotated on H&E only (N=60), and one using IHC-derived labels (N=46). For the HE-only GT, 3 pathologists annotated lymphocytes and all other cells within 4-5 fields of view (FOVs) per slide (277 FOVs). Consensus class was voted for labels within 3μm of each other. For the IHC-derived dataset, we de-stained the HE slides and re-stained them with an IHC stain for T & B lymphocytes (CD3 + CD20). Positively stained cells were detected within 5-10 FOVs per slide using QuPath (472 FOVs). These labels were then registered to the corresponding regions on the H&E where a pathologist corrected errors from QuPath and registration. An additional pilot dataset of 10 IHC slides was used to measure inter-pathologist variability on IHC, where 3 pathologists manually annotated lymphocytes in FOVs similar to what was done on H&E. A previously developed deep learning model trained on IHC-derived labels was evaluated on the FOVs from both datasets. The model predicts 3 classes: lymphocyte, other cells, background. For evaluation, we compute two F1 scores: cell detection (cell vs background), lymphocyte detection (lymphocyte vs other+background).
Results – Comparing GT on IHC pilot and H&E-only datasets, we find inter-pathologist agreement for lymphocytes is much higher when annotating on IHC (F1 0.75) than H&E (F1 0.58). Comparing AI model performance on IHC-derived and H&E-only datasets, we find the model overpredicts cells relative to H&E-only GT (% diff. 27.74 ), but agrees more closely with IHC-derived GT (% diff. 2.75) (Fig1). The model has improved cell and lymphocyte detection on IHC-derived GT (Table 1).

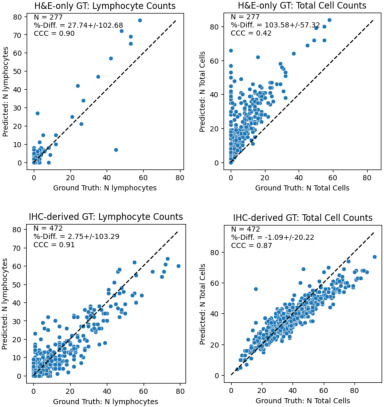
Conclusions – Lymphocyte detection model metrics are improved when measured against IHC-derived GT vs H&E-only GT because consensus annotations on H&E tend to under-label cells. This highlights the pitfalls of interpreting classification metrics from imperfect GT.
VIEW THE PUBLICATION