Background – Microsatellite instability-high (MSI-H) is a tumor-agnostic biomarker for immune checkpoint inhibitor therapy. Previous studies have shown that AI-based imaging predictors can infer MSI status from hematoxylin and eosin (H&E) whole-slide images (WSIs). We have developed AI models that predict MSI status in prostate, colorectal, and endometrial cancer and performed laboratory development test (LDT) validations following the CAP/CLIA standards for these models.
Design – H&E-stained WSIs of biopsies and surgical resections containing prostate cancer, colorectal cancer, and endometrial cancer were split into model development (prostate: n=4252, MSI-H 2.5%, colorectal: n=10445, MSI-H 6.7%, endometrial: n=2354, MSI-H 21%) and validation sets enriched for MSI-H (prostate: n=198, MSI-H 31%, colorectal: n=234, MSI-H 41%, endometrial: n=150, MSI-H 35%). Attention-based multiple instance learning models were trained to predict MSI status for each cancer type. For LDT validation, each model was evaluated for its analytical accuracy, analytical precision, analytical sensitivity, and analytical specificity with predefined acceptance criteria. The analytical accuracy study evaluated the model performance for predicting MSI status. The analytical precision study validated reproducibility and repeatability using inter-scanner and intra-scanner rescans of the same slides. For analytical sensitivity and specificity, we established a limit of detection (LoD) on the tumor area and a limit on the amount of blurring and color distortion in the images each model could tolerate.
Results – For analytical accuracy, the area under the receiver operating characteristic curve (AUC) was found to be significantly predictive of MSI status for all models (Table 1 and Fig 1). For analytical precision, the binary call concordance of the MSI-H prediction between the original scans and inter-scanner or intra-scanner rescans exceeded 80% at two target operating points for all models (Table 1). Table 1 also shows the LoD and maximum artifact percentages set by analytical sensitivity and specificity studies.

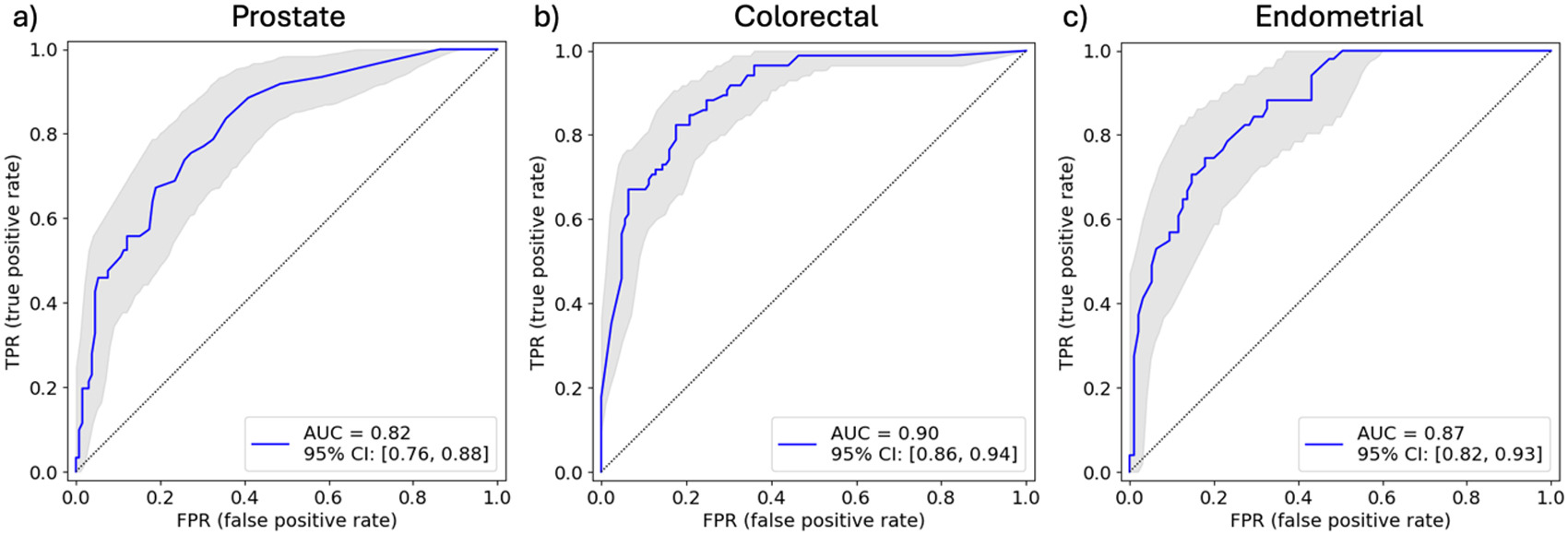
Conclusions – Our histogenomic models for predicting MSI status in prostate, colorectal, and endometrial cancer passed all acceptance criteria in their LDT validations. The prostate model is deployed internally with a pathologist in the loop to triage patients for mismatch repair proteins, immunohistochemistry confirmatory testing. More algorithm deployments will follow in the future.
VIEW THE PUBLICATION
VIEW THE POSTER